Individual to Mass Behaviour – I2MB
When a novel, highly infectious virus threatens the population, non-pharmaceutical interventions (NPIs) are needed to compensate for the absence of vaccines and medical treatments. However, NPI’s success is based on the individual changing their behaviour to accommodate the changes requested by the NPI [9], e.g., wearing masks and maintaining social distancing.
A commonly used NPI is contact tracing, which permits local health organisations to detect contacts of an infectious individual and isolate possible contacts at risk. However, given the resources of local health authorities and the increasing population density of urban areas, manual contact tracing (MCT) is insufficient to control the pandemic effectively [3]. In the case of the COVID-19 pandemic, digital contact tracing (DCT) was introduced to identify and isolate possible contacts between susceptible and infectious members of the population. Theoretically, DCT has the potential to scale at the level of current population densities and overcomes the limitations of people remembering the time and place of an event [1].
To get ahead of the COVID-19 pandemic, researchers are using two families of models: compartmental models and agent-based models (ABM). Compartmental models, like the SEIR models, incorporate the effects of NPIs as additional dynamic terms in the differential equation or as modifiers to the dynamic rates of state changes [4]. Compartmental models are ideal for simulating large populations, e.g., entire countries. However, they cannot represent the population’s geographical and ideological clustering [2, 5]. ABM models are used to analyse interactions at more local scales, e.g., medium-size cities. ABM covers individuals’ interactions and is usually limited to home, office, and random interactions [7]. However, random interaction networks do not represent the clustering generated by routines, where people are not at home but have consistent, albeit with noise, interaction networks [8].
We hypothesise that an ABM based on behaviour modelling provides the missing dimension to accurately predict the effect of NPIs at the point in the pandemic where no data has been observed. Furthermore, NPIs like social distancing and DCT have a strong dependence on the physical position of individuals during the interaction. Therefore, we propose the Individual to Mass Behaviour (I2MB) simulation toolbox, a behaviour driven, physically positioned, agent-based simulator.
Our Methodology
I2MB is a modular agent-based simulation engine written in python. The architecture is illustrated in the following image. Each module provides functionality that modifies the state of the environment or the agents within them. We diverge from most ABMs that use a probabilistic infection transfer model. Instead, we implemented a viral load transfer module that uses exposure time, distance and viral load concentration in the host to simulate a more mechanical transfer of the pathogen between agents. The viral load transfer model implements a recovery function that opposes viral load accumulation in the exposed individual, simulating the body’s natural defence mechanisms. Once the viral load reaches a self-sufficient level, the exposed agent enters the infected state, and viral load starts to develop following an incubation time and a maximal load characteristic of the disease. A gamma function, parameterised by the maximal viral load and incubation period, is estimated to control the agent’s viral load at each simulation step.
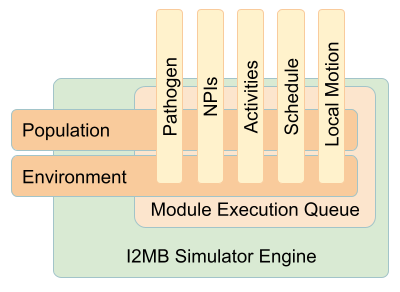
Our strategy for behaviour simulation uses a hierarchical approach. The top behavioural layer determines the agent transition between spaces, equivalent to people’s daily schedules. An intermediate layer defines location-specific behaviours, like sleeping, cooking, eating, showering when agents are at home, and working when the agents are at the office. At the lowest layer, a random walk module represents the agent wandering about while doing nothing or activities like cleaning, where the agent’s position could be described as a random walk. We have built a virtual world for agents to live in. Therefore, we can simulate NPIs like closing bars and restaurants. The environment is a part of the simulation. It is modelled by dynamic components created to modify the random walk or provide opportunistic behaviours in the simulation, e.g., sit or stand, depending on the seat availability.
With an agent’s physical position and a complex world, we can simulate different NPIs. We chose to evaluate DCT taking advantage of the physical distance provided by the other modules. With physical distance and location between agents, various mobile phone technologies, e.g., GPS or Bluetooth, can be approximated to effectively evaluate the effect of DCT applications like the CoronaWarnApp (CWA). Furthermore, we look at the impact of closing restaurants and bars and use a behavioural approach to determine if people would follow advice like self-isolating at home, getting tested, and quarantining the entire household.
Our Evaluation Strategy
Our simulation has an extensive parameter set. To avoid expensive parametric searches, we set most module parameters based on well-known literature. Furthermore, our virtual world is not modelled after any particular city. Provided the number of agents in the simulation, things like public transport utilisation and availability are influenced by statistics from the real world. However, the emerging behaviour by the superposition of modules does not have a direct counterpart in literature. Therefore, we do digital experiments to measure the same statistics reported in the literature. For example, we calculate the average time agents spend performing a specific activity in the simulation and compare those results to reported values.
Our evaluation strategy is split into two parts: 1) we verify that the simulator can reproduce reported statistics based on activity durations, the number of contacts generated, serial and generation intervals, and pathogen reproduction numbers. 2) We introduce different combinations of NPIs and evaluate their effect on wave duration, the number of deceased and susceptible agents remaining at the end of the wave, the peak hospitalised agents and the peak maximum infected. The NPIs under evaluation are the following: DCT and MCT strategies, testing negative before leaving isolation, 14 dais quarantine or self-isolation, and household quarantine of primary case and contacts.
Preliminary Validation Results
We created a virtual world where each agent was assigned a home and an office place to validate our approach. In the following video, we illustrate simple routines like sleeping and working. Furthermore, we show agents going to the restaurant or the bar. The video depicts a successful run of a small population where the infectious individual was isolated just in time, and the epidemic did not evolve across the population.
We measured serial intervals in our sample population of 30 agents and noticed that the serial interval distribution was close to the serial interval distribution measured in actual patients reported by He et al. [cite PRTP9654]. We are investigating the effect of detailed indoor activities on the serial interval distribution.
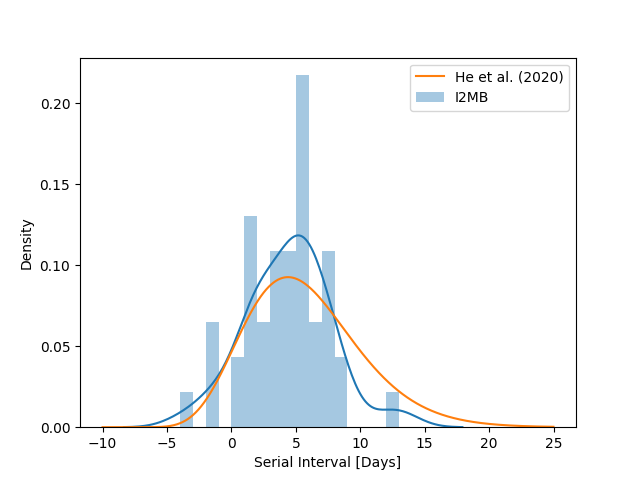
Using contact duration as a parameter, we built a contact network. We found the contacts clustered around homes or offices with some interconnections caused by spurious meetings at restaurants or bars. The following figure illustrates a network where the primary clustering is caused by office connections.
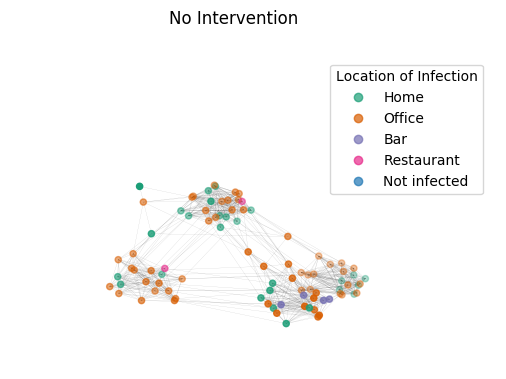
We built infection propagation chains to compute the initial reproduction rate R0. In the case of the chains shown in the following figure, the initial reproduction number was 7.4, which is at the high end of reported values. However, given the small population size, the chains stopped after three generations affecting the entire virtual population.
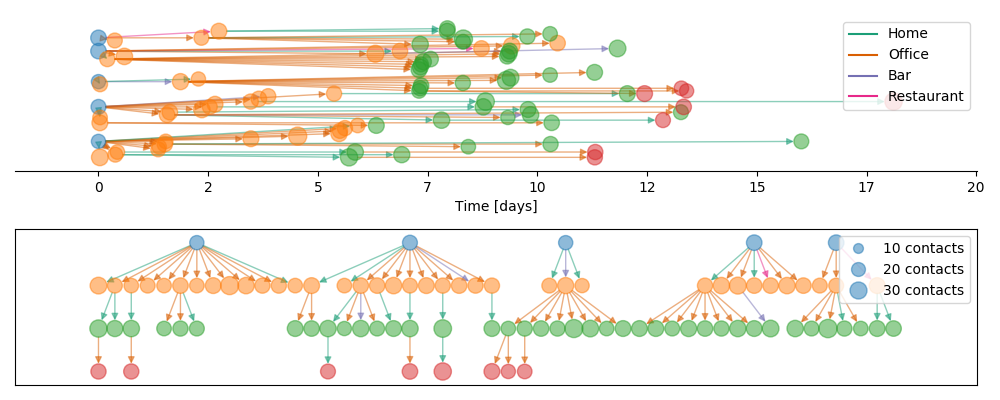
Contributors and Partners
The project is a collaboration between CDH and the Robert-Koch Institute (RKI).
FAU-CDH
Luis I. Lopera
Oliver Amft
Student Contributors
Miriam Kraft
Laura N. Hennig
Jad Kassam
Katarina Marceta
RKI
Justus Benzler
Göran Kirchner
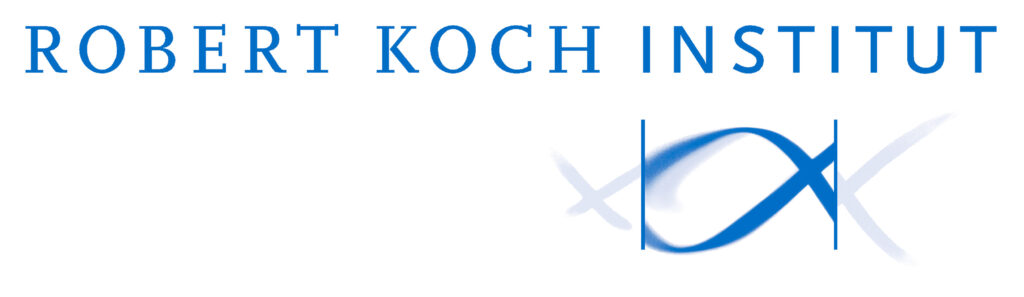
References
- Oliver Amft, Luis Ignacio Lopera Gonzalez, Paul Lukowicz, Sizhen Bian, Paul Burggraf, “Wearables To Fight COVID-19: From symptom tracking to contact tracing“, IEEE Pervasive Computing, 2020.
- Jacques R. Bughin, Michele Cincera, Dorota Reykowska, Marcin Żyszkiewicz, Rafal Ohme, “Perceptive Risk Clusters of European Citizens and NPI Compliance in Face of the COVID-19 Pandemics“papers.ssrn.com, 2020/12/16.
- Luca Ferretti, Chris Wymant, Michelle Kendall, Lele Zhao, Anel Nurtay, Lucie Abeler-Dörner, Michael Parker, David Bonsall, Christophe Fraser, “Quantifying SARS-CoV-2 transmission suggests epidemic control with digital contact tracing“, Science, science.sciencemag.org, 2020/05/08.
- Christophe Fraser, Steven Riley, Roy M. Anderson, Neil M. Ferguson, “Factors that make an infectious disease outbreak controllable“, Proceedings of the National Academy of Sciences, www.pnas.org, 2004/04/20.
- Federico Germani, Nikola Biller-Andorno, “The anti-vaccination infodemic on social media: A behavioral analysis“, PLOS ONE, PLoS Journals, 3 Mar 2021.
- Xi He, Eric H. Y. Lau, Peng Wu, Xilong Deng, Jian Wang, Xinxin Hao, Yiu Chung Lau, Jessica Y. Wong, Yujuan Guan, Xinghua Tan, Xiaoneng Mo, Yanqing Chen, Baolin Liao, Weilie Chen, Fengyu Hu, Qing Zhang, Mingqiu Zhong, Yanrong Wu, Lingzhai Zhao, Fuchun Zhang, Benjamin J. Cowling, Fang Li, Gabriel M. Leung, “Temporal dynamics in viral shedding and transmissibility of COVID-19“, Nature Medicine, www.nature.com, 2020-05.
- Robert Hinch, William J. M. Probert, Anel Nurtay, Michelle Kendall, Chris Wymatt, Matthew Hall, Katrina Lythgoe, Ana Bulas Cruz, Lele Zhao, Andrea Stewart, Luca Ferritti, Daniel Montero, James Warren, Nicole Mather, Matthew Abueg, Neo Wu, Anthony Finkelstein, David G. Bonsall, Lucie Abeler-Dorner, Christophe Fraser, “OpenABM-Covid19 – an agent-based model for non-pharmaceutical interventions against COVID-19 including contact tracing“, medRxiv, www.medrxiv.org, 2020-09-22.
- Giancarlos Troncoso Parady, Genki Katayama, Hiromu Yamazaki, Tatsuki Yamanami, Kiyoshi Takami, Noboru Harata, “Analysis of social networks, social interactions, and out-of-home leisure activity generation: Evidence from Japan“, Transportation, Springer Link, 2019-06-01.
- Talya Porat, Rune Nyrup, Rafael A. Calvo, Priya Paudyal, Elizabeth Ford, “Public Health and Risk Communication During COVID-19—Enhancing Psychological Needs to Promote Sustainable Behavior Change“, Frontiers in Public Health, Frontiers, 2020.
Contact
Dr. Luis I. Lopera G.
- Job title: Researcher
- Address: Henkestraße 91, Haus 7, 1. OG
91052 Erlangen
Germany - Phone number: +49 9131 85-23605
- Email: luis.i.lopera@fau.de