Eating timing estimation
An emerging number of methods are proposed for eating event detection. To assess the detection performance, evaluation metrics such as precision, recall and F1 score are commonly used. However, these popular metrics only give overview of event retrieval performance and do not tell people the absolute timing error of the eating detection algorithm. Meanwhile, the eating timing error is an important marker for applications. For example, diabetic patients can manage the insulin injection time better with a preciser detected eating timing. We propose using eating timing errors, i.e. the absolute time differences at the eating start/end between an detected event and ground truth, as evaluation metrics for eating detection.
Eating spotting methodology
As an example, we estimated the eating timing errors of our eating spotting algorithm based on a one-class Support Vector Machine (ocSVM). We collected bilateral Temporalis electromyography (EMG) data from 10 participants in free-living using smart eyeglasses integrated with EMG sensors. A feature vector of only 6-dimensions were extracted and fed to the ocSVM spotter. We varied the spotting window size w with/without a fixed overlapping section length of 1 second. For the evaluation, leave-one-participant-out (LOPO) cross-validation was used.

Eating spotting procedures investigated.
Eating spotting results
The result showed that the highest F1 score is not always related to the lowest timing errors. The lowest timing errors (mean ± std.) were achieved at different window sizes: 21.8 ± 29.9 seconds for the eating start, and 14.7 ± 7.1 seconds for the eating end.
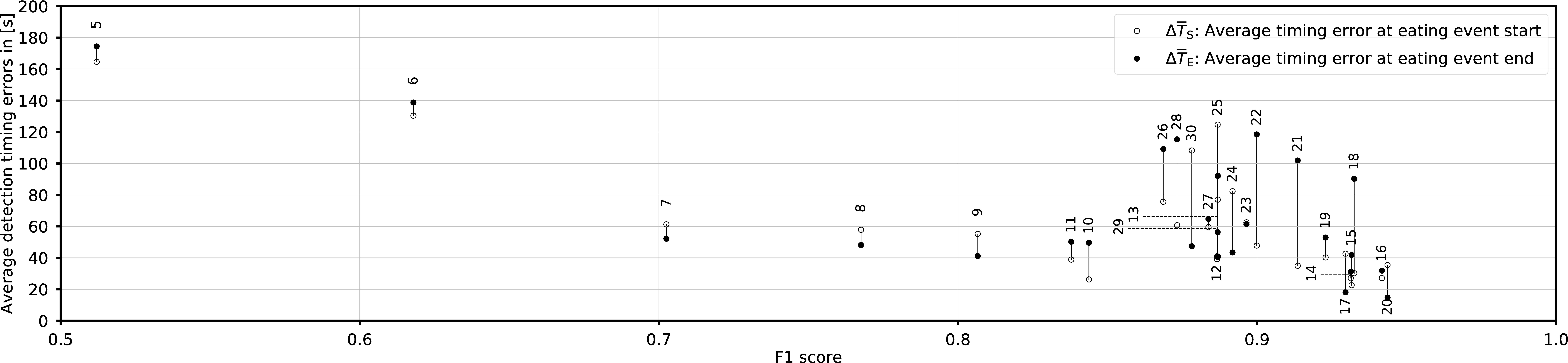
Average spotting timing errors vs. F1 score in non-overlapping window mode: Markers connected by a solid line correspond to identical window length w. Value of w is annotated next to the marker pair.
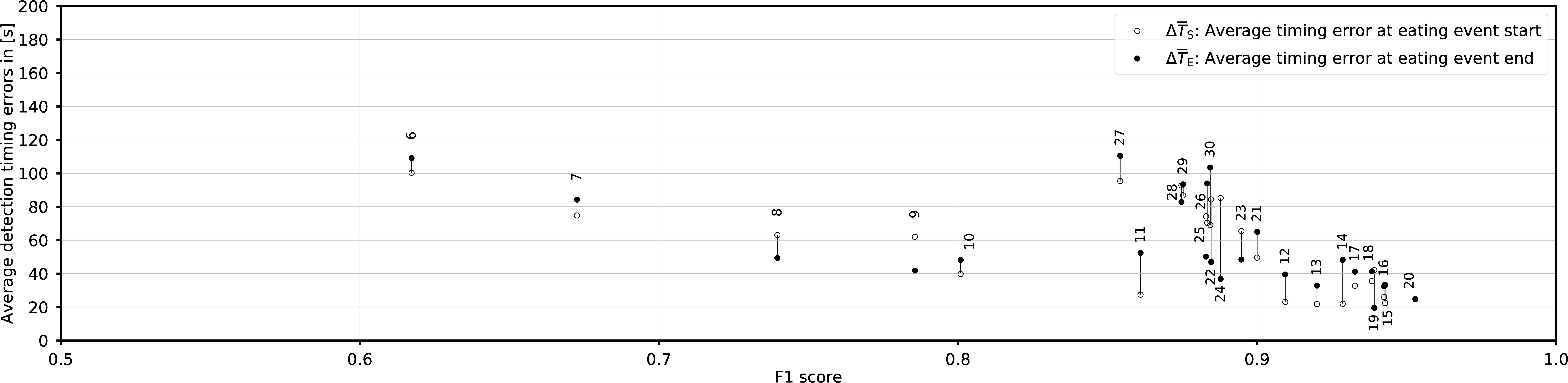
Average spotting timing errors vs. F1 score in overlapping window mode: Markers connected by a solid line correspond to identical window length w. Value of w is annotated next to the marker pair.
Related projects
- WISEGlass
- Chewing monitoring
- Automatic dietary monitoring
Publication
[publication id=”JKE9TZUC”]
[publication id=”CMGF8KAU”]
Contact
Rui Zhang
- Job title: Researcher
- Address:
Henkestr. 91, Geb. 7
91052 Erlangen - Phone number: +49 9131 85-23604
- Email: rui.rui.zhang@fau.de